IoT
Industrial IoT predictive maintenance
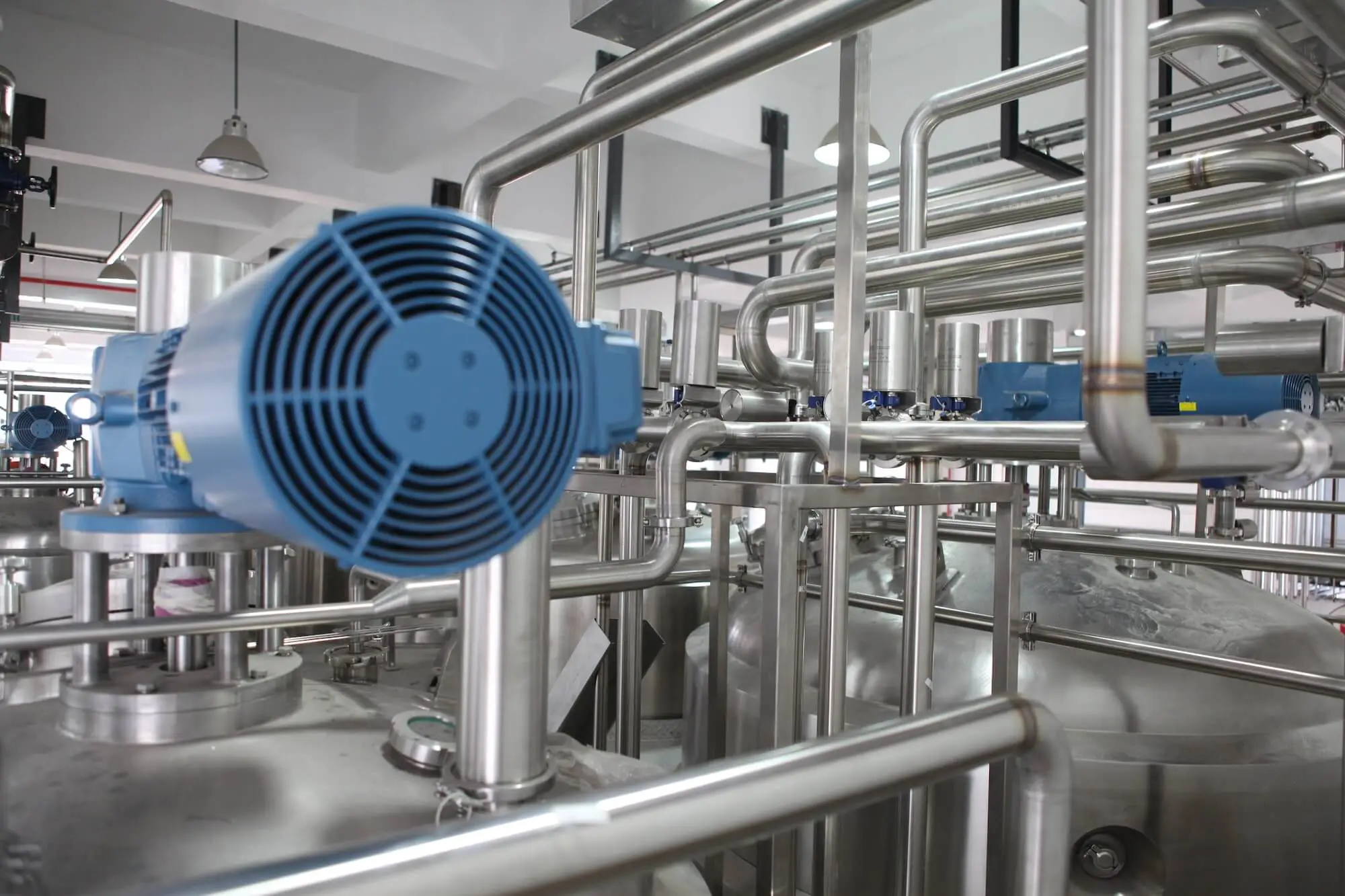
What is industrial IoT predictive maintenance, how is it used, and what are the benefits of choosing predictive vs. reactive maintenance?
Industrial IoT predictive maintenance provides one of the most critical business optimization opportunities available with Industrial Internet of Things technologies by moving from lifecycle-based or scheduled maintenance to condition-based maintenance.
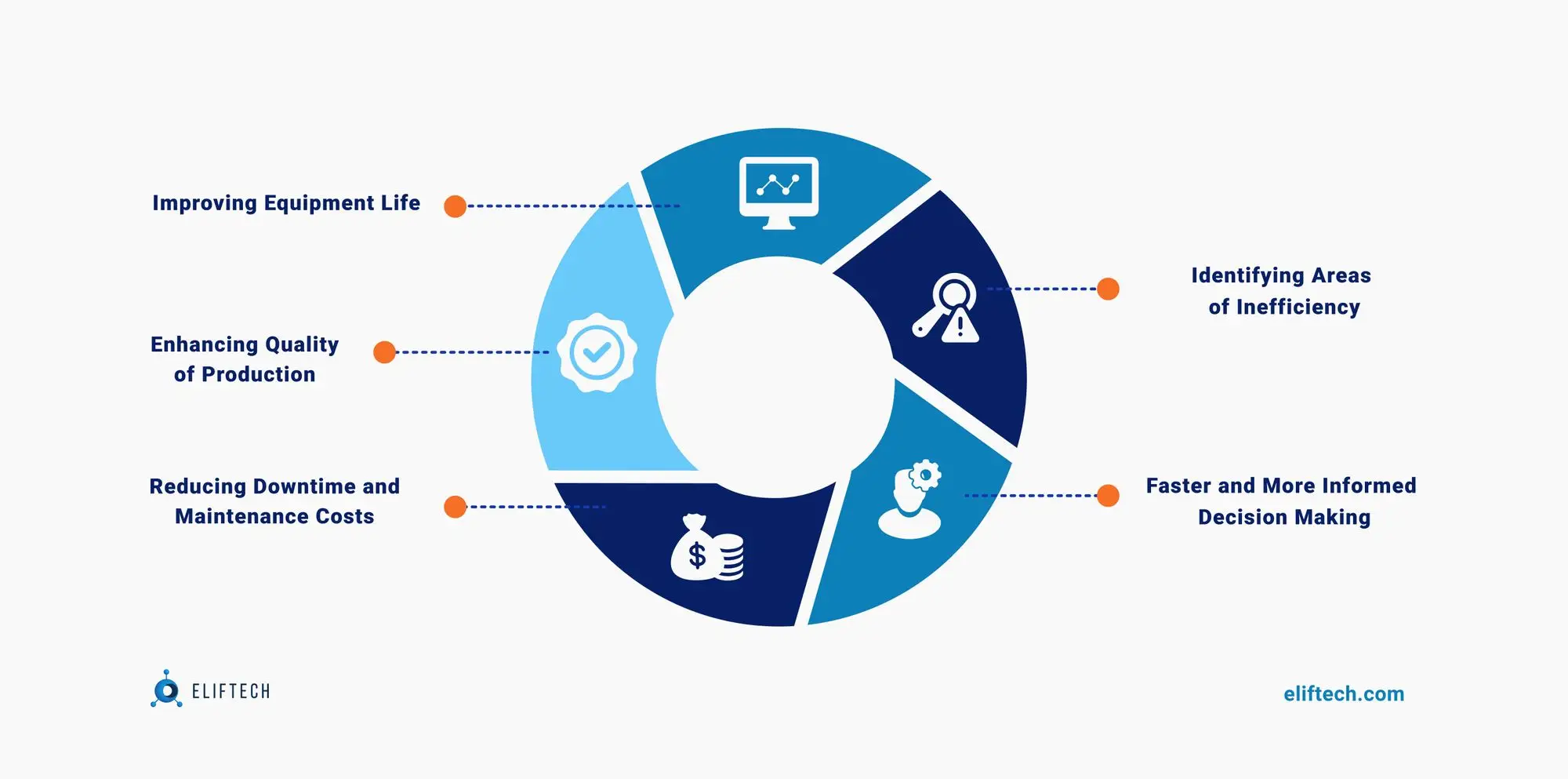
Preventive maintenance can be used to avoid unplanned repairs and reduce costs. Regular medical equipment checks ensure its best functioning. This type of maintenance collects real-time data and relevant device data from multiple data sources. The IoT devices are equipped to collect sensor data that experts can analyze to determine what services are needed. IIoT predictive maintenance business value lies in the increased equipment life, the possibility to predict equipment health and lower operating costs due to less frequent repairs, and stricter Service Level Agreement (SLA) rules.
What is predictive maintenance?
Industrial enterprises consist of assets of several types. The sensor-based Internet of Things is used to diagnose and predict asset behavior. For example, rotating parts of machinery are often subject to mechanical wear. Failure to monitor this wear and tear can lead to machine breakdowns and unexpected plant shutdowns. In addition to mechanical faults, machines can also experience electrical faults. Therefore, monitoring the condition of these machines is very important for the early detection of defects to avoid unscheduled repairs, minimize downtime, and thus guarantee the devices' reliability, uptime, and stability. Several non-invasive methods of monitoring the machine's condition can be performed with the help of sensors. Most are based on measuring machines' current, vibration, and acoustic emission. Converters are needed to receive these signals. Remote monitoring requires wireless connectivity and IoT pipelines.
Condition monitoring of equipment - both electrical and non-electrical - is one of the main requirements for Industrial IoT Industry 4.0. Although the sensor-based data-driven solution looks like a machine-learning problem, it is impossible to solve the issue of component-level diagnostics with machine learning. For example, sensory equipment data can be noisy, and depending on the signal-to-noise ratio, the traditional machine learning approach will train noise instead of the desired signal itself. Another problem is that time domain data signatures cannot distinguish, isolate or understand the underscore problem with a machine without signal transformations such as Fourier, wavelets, time-frequency, Hilbert, etc.
Using the Fourier transform of a signal, we can display many signatures that point to a particular anomaly in the machine. Because the patterns have different characteristics, this approach is unsupervised and does not require any historical data to generate the output.
How to use DSP for AI/ ML as IIoT predictive maintenance tools
There are two ways to use DSP.
- The first one is DSP-based data conversion. This methodology is used in the capacity of an ETL tool. The transformed data is used as another feature of the dataset. Subsequent ML/AI blocks consume data in a statistical sense and learn underscore patterns to draw conclusions. Most data scientists choose this approach, but in many cases, it is suboptimal because there is no domain definition and no understanding of the transformations.
- The second is the DSP-Based Design and Pinouts. In this method, the data is pre-exposed/converted to another area by DSP, but knowledge of the DSP area is required to generate inferences that are further used to improve top-down AI/ machine learning algorithms. A deep understanding of signals and systems is a prerequisite for building the best machine-learning models. This method is a must in cases where it is necessary to diagnose the internal components of assets and not just trends. For example, the gear-to-gear problem cannot be detected with raw machine learning or DSP like ETL+ML. We need to understand what harmonics mean, what energy in the frequency band means, the noise level, attenuation, phase relationships, the ability to distinguish the sensor signal from industrial noise, and the like. This approach can be applied to almost all asset classes – motors, pumps, compressors, generators, conveyors, etc.
DSP is followed by feature engineering, during which features are extracted from the transformed data. It is performed in addition to the original tasks from the dataset, which provide a deep understanding of the data that would not otherwise be possible. The process is then split into two. First, in a domain-specific way, we infer signatures resulting from multiple transformations from a domain perspective and derive a diagnostic model based on them. Further, extended model training with diagnostic signatures can train AI predictive models to obtain RUL and other predictive maintenance-related metrics. The second way is the statistical inference approach, which provides additional information for model building, but is purely statistical.
What is the role of IIoT in predictive maintenance?
The most straightforward way to explain preventive maintenance's advantages is with specific, real-life examples. Imagine a college campus. The technical maintenance crew keeps the hot water running during winter break to prevent pipes from freezing. Dreading a scenario where the pipelines break under cold temperatures is natural, especially if the building is unoccupied when the leaking or breakage occurs. It is difficult to access such leaks, and they may need heavy equipment to lift large pipes for repair.
Now imagine campus maintenance crews installed in-pipe sensors to monitor system-wide water-flow rates. Instead of zeroing in on a leak based on data from the usage meter, the team went for the IIoT. Their choice is a demonstration of forward-thinking, and it is far more precise. This case vividly illustrates how carefully chosen, and correctly placed sensors allow campus crews to get the data to detect and repair small leaks long before they become major problems.
Let's take that one step further. Following similar data-collection principles, it is possible to use predictive analytics to derive actionable insights into the overall system's health and replace the components before they wear out. This insight would allow for replacing separate damaged components of the system at a fraction of the cost instead of performing repairs after the critical failure.
Just in case you didn't know, we currently live in a world where water systems are normally expected to lose up to 50% of their payload to leaks. This means we cannot overestimate the value of preventing leaks and other breakages. However, it takes a vast amount of reliable data and powerful predictive analytics software to achieve the necessary efficiency.
To apply this approach in remote or hard-to-access locations and consistently save critical assets, time, and money, it is necessary to use the IIoT. The most modern sensors can report their health and status, for example, battery life.
With solid data and proper predictive analytics, preventive maintenance may be timed for maximum uptime and efficiency. Important note: the number of sensors isn't everything. Although the sensors are vital, they are only a part of the preventive maintenance landscape. For raw data to have significance, it must be analyzed. The analysis results can, in turn, inform better business decisions.
How industrial IoT predictive maintenance works
In many cases, implementing predictive maintenance software is not even an option, as the telemetry received from the equipment is the main argument in settling disputes between the equipment supplier and the operator. The agent built into the equipment can work autonomously, allowing the supplier to meet the requirements for temperature, humidity, slope, overload, and other parameters during transportation and storage.
When implemented correctly, IoT predictive maintenance solutions evaluate two important indicators of device health: Time to Failure (TTF) and Remaining Useful Life (RUL). These metrics are easily converted into work cycles, kilometers driven, the number of transactions, and other parameters. Remaining life estimation is a technically difficult problem, but it works well for rotating machinery such as fans, pumps, and motors.
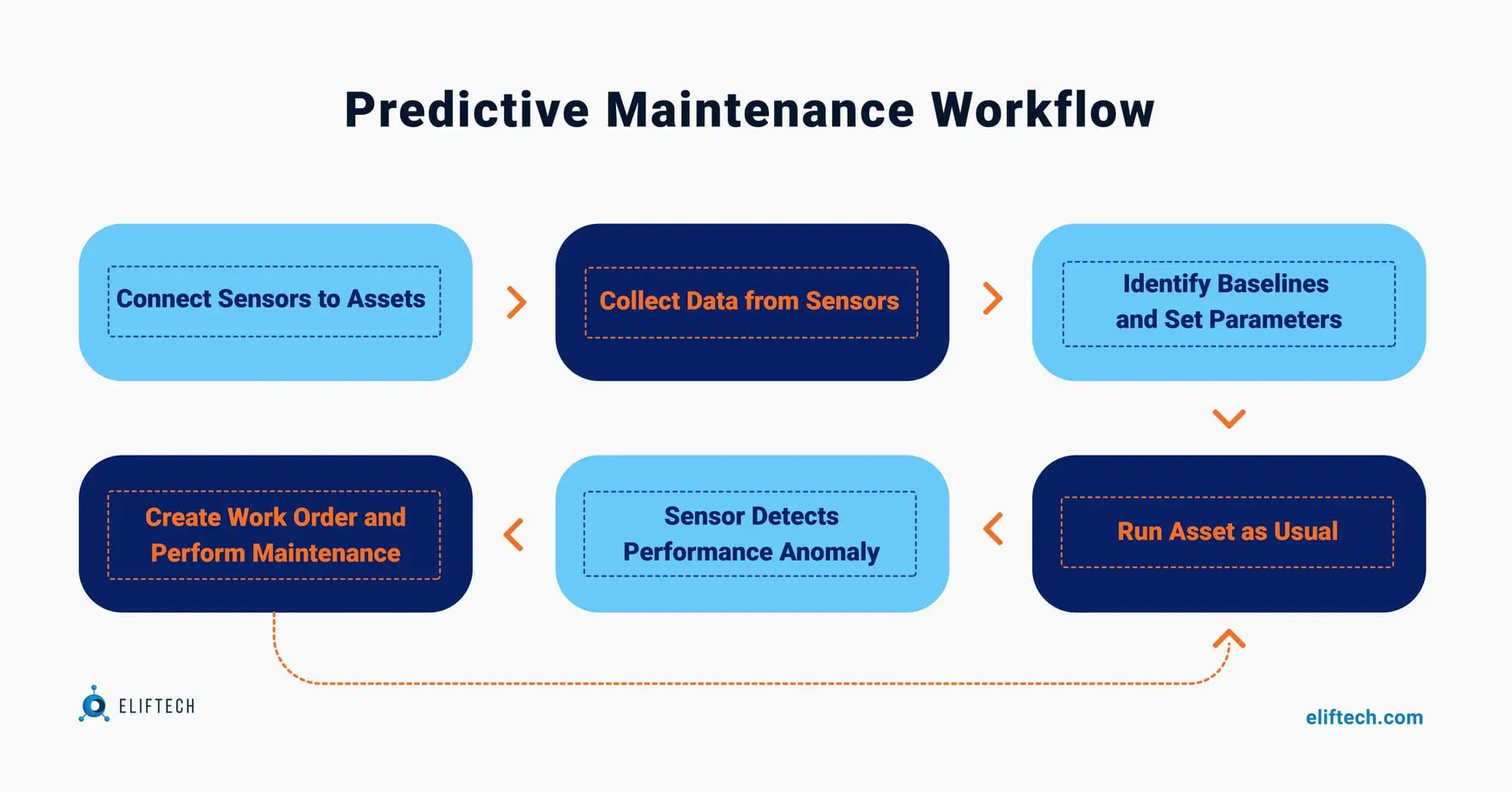
IoT-based predictive maintenance is typically integrated with a computer-aided maintenance management system (CMMS) that automatically generates equipment deterioration requests. Another leading function is the logistics of the service. A proper IoT-based predictive maintenance solution has in-depth knowledge of the specific operation of the equipment and uses it to tune and optimize the life cycle and control logic intelligently.
Benefits of IIoT predictive maintenance
Businesses can save 12-18% through preventive maintenance. Moreover, unscheduled maintenance is very demanding. Early statistics show that 80% of employees waste an average of 30 minutes per day retrieving information. When something goes wrong (and it always does), employees will spend even more time locating and understanding the issue. The bigger the business's operation, the greater the time drain, and the more it affects your budget. It is worth noting that the mentioned Forbes article focused on predictive maintenance for manufacturing at the time of the survey.
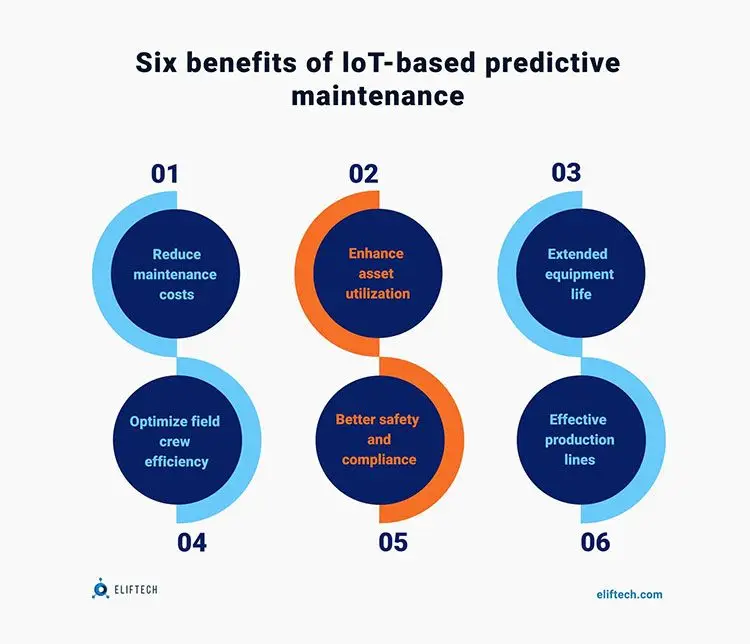
Sadly, the sheer number of field service technicians (more than 20 million such workers worldwide) indicates the scale of the potential savings for preventive and predictive maintenance. By now, a vast number of manufacturers dispatch technicians to tend to heavy machinery in an attempt to collect data "manually" because the equipment isn't powered by IIoT. Implementing an IIoT predictive data gateway to collect relevant data on already-existing equipment saves time and money. Plus, it consistently provides businesses with the data they need to unlock significant cost savings. Furthermore, you will no longer need to dispatch a maintenance worker, who is often required to drive long distances to a remote location to gather information from a device that may normally be operating. The Modern IIoT system allows your technicians to review information without the actual need to travel.
Once your IIoT system is set in place, you only need to dispatch technicians when preventive maintenance measures are required. On top of that, for many devices, issues can be solved or corrected remotely. For example, pumps can be taken offline even before the damage occurs. The Forbes piece noted that by switching from "fix as you go" to an IoT-based predictive maintenance model, manufacturers expect a 78% jump in service calls requiring no "on-site" attention, meaning that they could be diagnosed and repaired remotely. They will save a great deal of time and money by avoiding the costs associated with unnecessarily dispatching a field technician.
Steps to start IIoT predictive maintenance
Modern IIoT ecosystem provides a short turnaround time for an IoT-based predictive maintenance system. This is what a typical project lifecycle looks like:
- Your machinery is equipped with various sensors to enable telemetry data collection.
- The “brain” of your hardware (usually programmable controllers or industrial PCs) is upgraded with a small piece of software called “the agent.” The agent monitors the receipt of all data collected by a central server installed in a public or private cloud.
- If editing the existing firmware is impossible, an external agent (a small programmable controller) can be added to this control computer. This external agent can connect directly to the sensors or receive data from the host.
- Data is seamlessly transferred to the server and stored in a high-performance database. In quick deployment scenarios, it is also possible to import historical data.
- Once enough data has accumulated, it is necessary to show the system which behavior patterns of the installation should be considered negative.
Further processing is done without the participation of users and system administrators. Predictive maintenance involves applying machine learning technologies and big data analysis algorithms to forecast equipment failure and deterioration.
Conclusion
Most industrial IoT systems today have well-thought-out maintenance programs. However, many plant managers still ask themselves what is better for the IIoT system - preventive or predictive maintenance. Both types of maintenance should be implemented to get the most out of it. This often raises additional questions: why should we continue to use old technologies? Shouldn't we use new technology and only preventive maintenance?
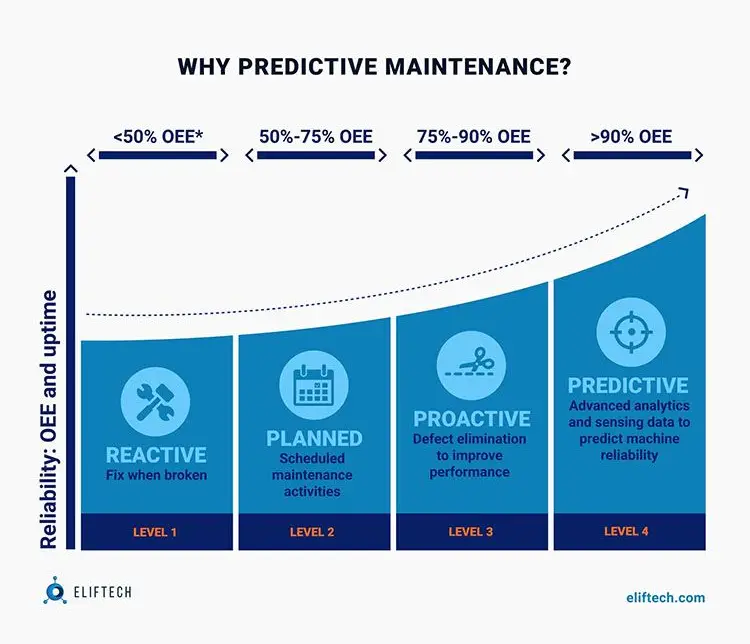
Predictive maintenance is the future wave, and new technologies will help industrial customers, business leaders, and owners make data-driven decisions. For example, alerts will be configured into analytics programs to notify users when certain metrics are out of range. When this happens, maintenance personnel can use all the data from the IIoT system to best implement strategies to eliminate possible causes of failure and plan downtime for maintenance. Either way, factories can shut down certain lines at scheduled times, saving business owners money for maintenance staff. Preventive and reactive maintenance is still appropriate for many IIoT systems. However, while predictive maintenance is the future trend and will help improve uptime, it won't work for every system. For example, the control cabinet, control panel, and switchboard components have critical equipment.
Looking for technical expertise to power your IoT system with a predictive maintenance model? Contact our team for a consult.
Considering embedding a predictive maintenance model within your IIoT ecosystem? Turn to our IoT teams for help!